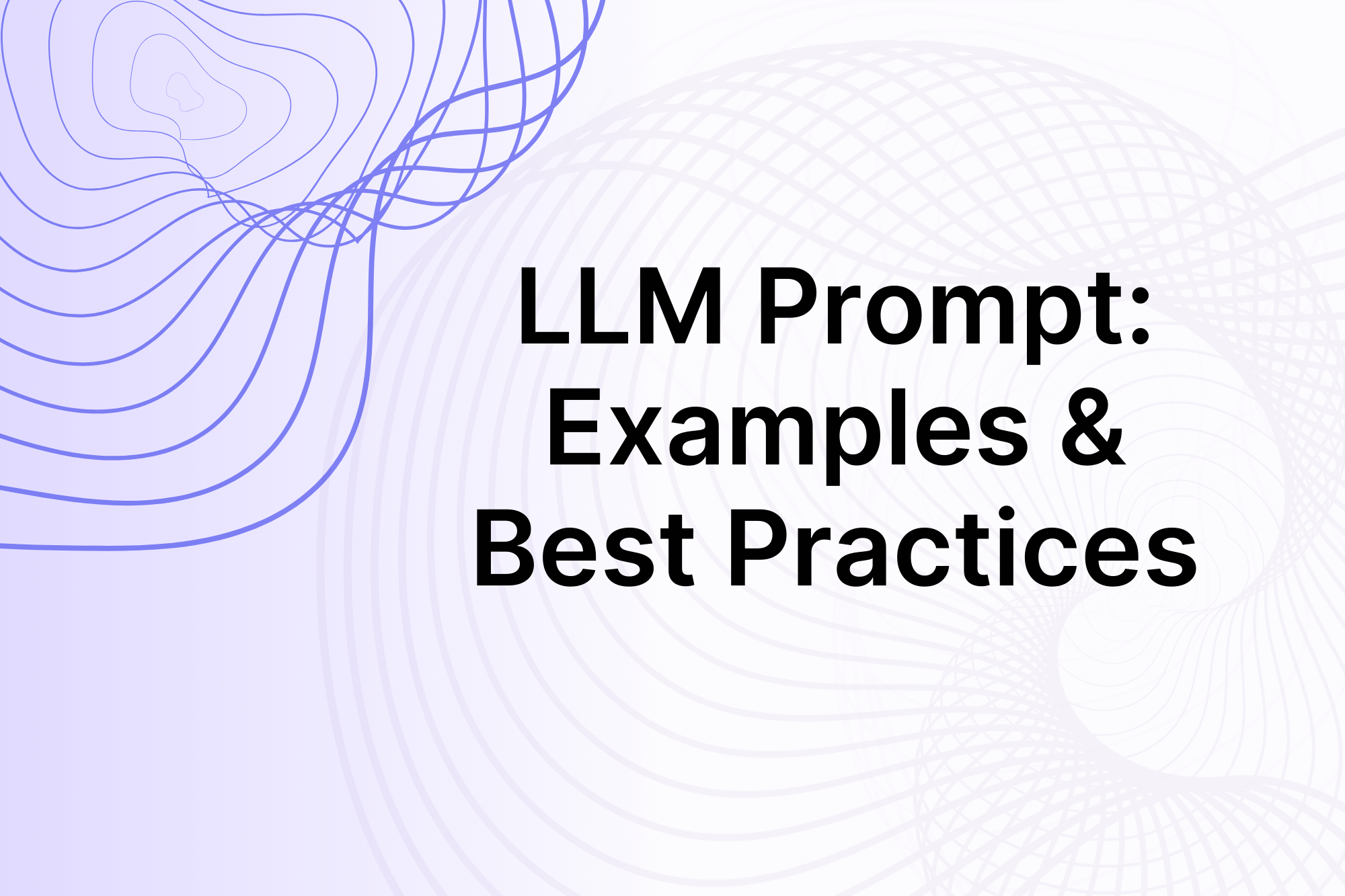
LLM Prompt: Examples and Best Practices
Everything you want to know about LLM prompts, including examples, tips, and coding examples for creating well-structured and effective prompts.
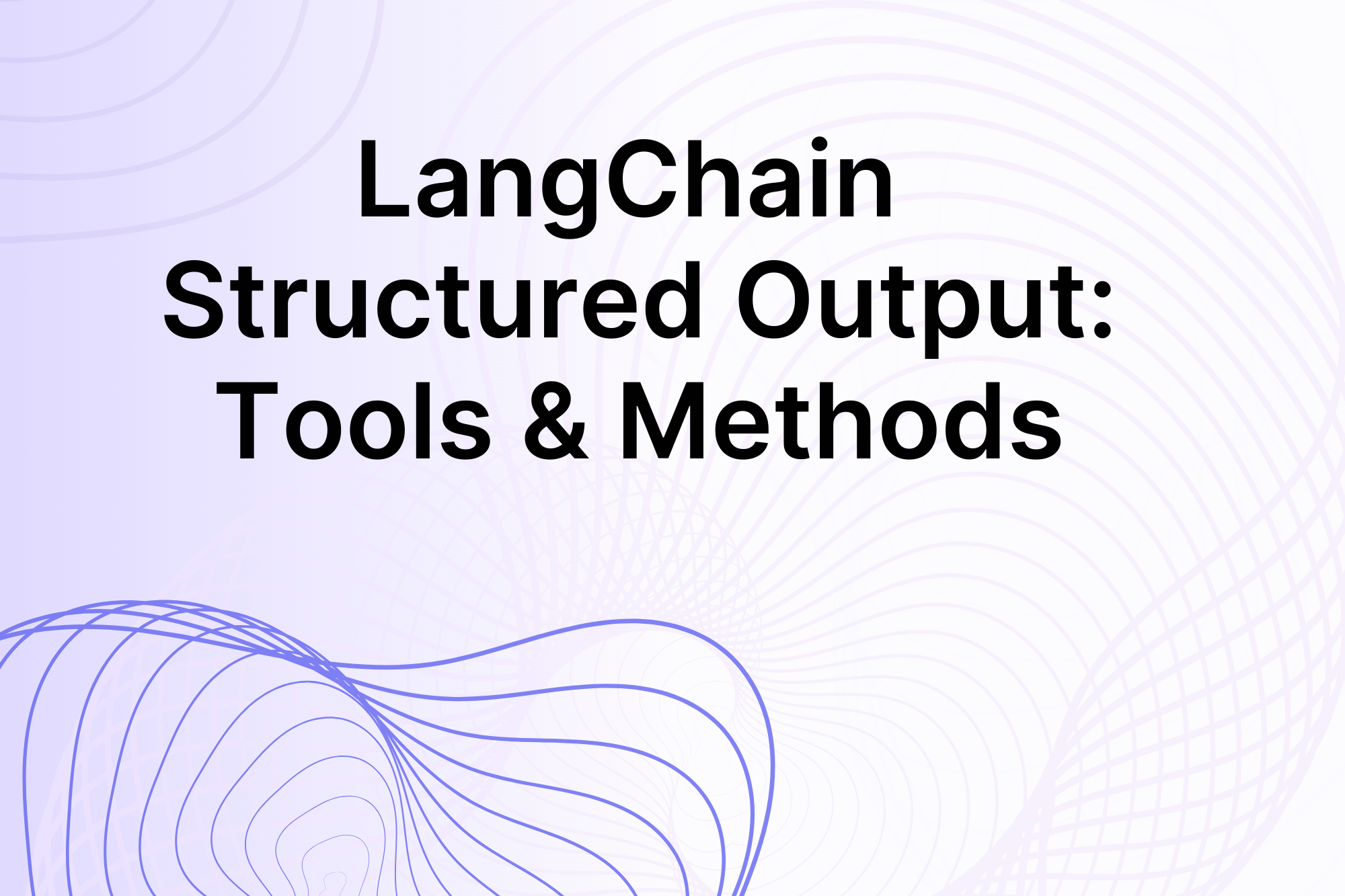
LangChain Structured Output: A Guide to Tools and Methods
This guide covers how to work with LangChain structured output and showcases how Mirascope provides an accessible and flexible solution for developers.

LLM Applications: What They Are and 6 Examples
Curious about LLM applications? Discover what they are and see 6 practical examples of how large language models are being applied across sectors.
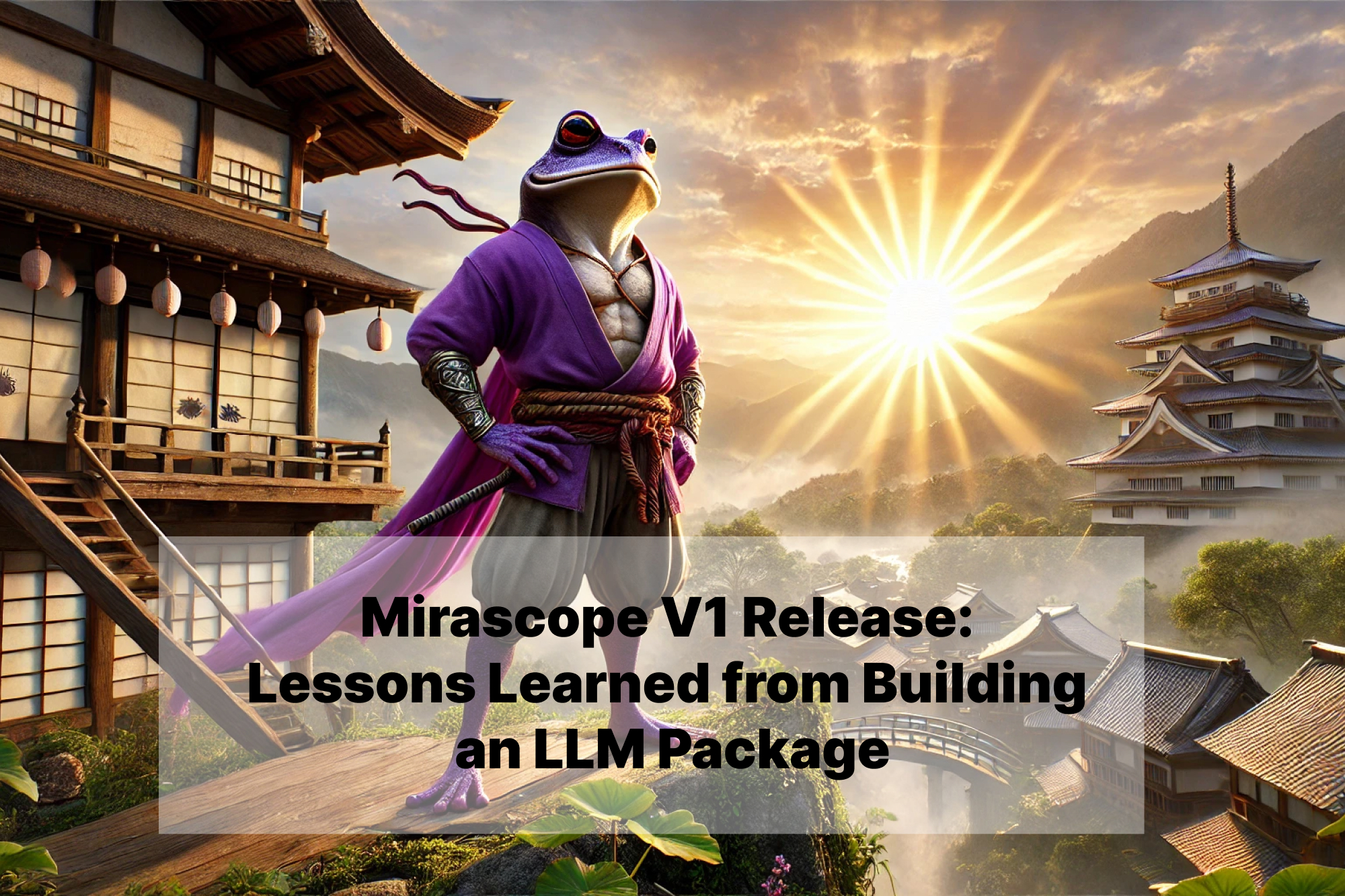
Mirascope V1 Release: Lessons Learned from Building an LLM Package
Mirascope's stable release, V1 has been released. We discuss the reasons behind creating Mirascope and what led us to the changes from the original design to our V1 and what's coming down the road.
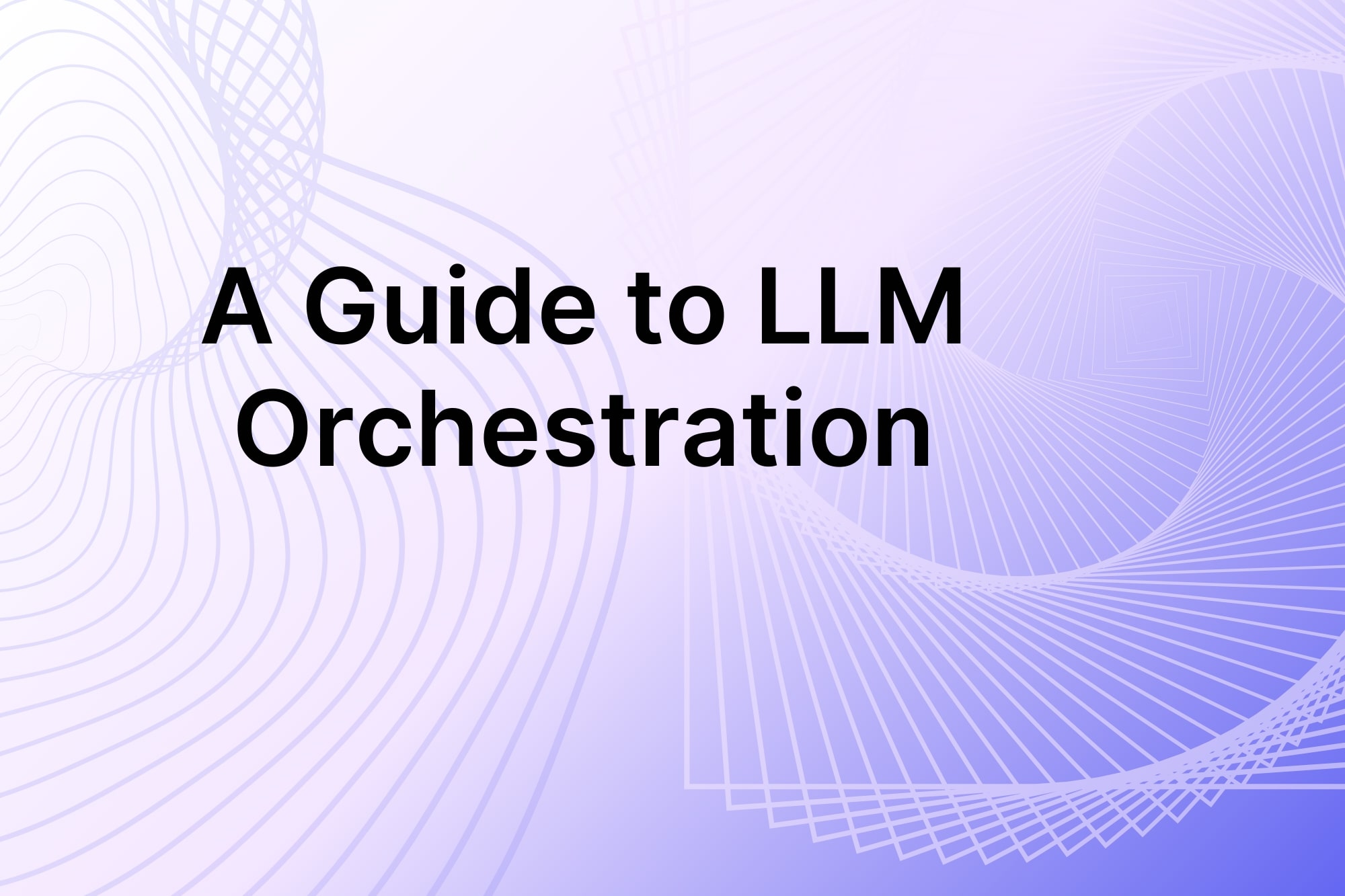
A Guide to LLM Orchestration
Explore our detailed guide to LLM orchestration and discover how it improves the performance and efficiency of applications driven by large language models.

Building an LLM Pipeline: Tools and Techniques
Learn how to build an efficient LLM pipeline, focusing on effective data handling and query generation techniques for retrieval augmented generation.